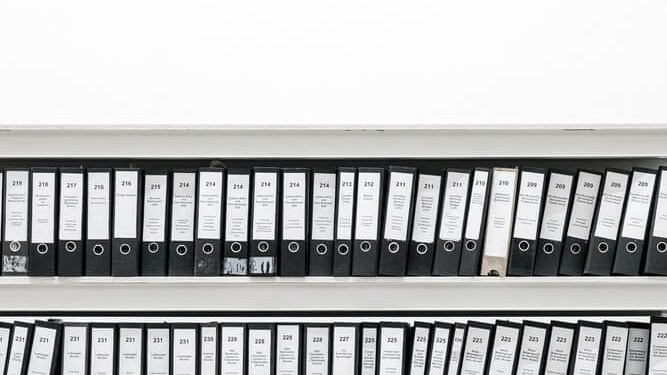
how machine learning can be used to manage a branch network
by Alexander Reiss
Automotive companies, fast food chains or any company operating a large branch network – especially at international level - all face the same challenge: how to manage these networks as efficiently and impartially as possible. We give answers on how artificial intelligence may be the solution.
Managing a branch network by using artificial intelligence
Time-eaters: KPI processing and interpretation
Often the approach is to draw-up a very large number of key performance indicators as a means to assess the performance of individual branches. The process of reading, aggregating and visually processing the data takes an enormous amount of time, but is necessary to present the results to the Management Board. The Board uses this data to assess performance of individual branches and devise subsequent strategic measures. All in all, a time-consuming process.
Consider this scenario:
There are five board managers on the final Management Board who set strategy for the branch network. They each take an average of five minutes to discuss a single branch. This means 25 minutes per branch for all five board managers. If the company operates a network of 5,000 branches, the board needs 125,000 minutes of management time per control cycle. This corresponds to 2,083 working hours – more than the entire annual working time of an employee working a 40-hour week!
By intelligent (partial) automation of this process, valuable management work time can be released with a manageable project effort.
Simplifying the control process through artificial intelligence
The above scenario is a good case for applying artificial intelligence to optimize the control process. An AI-based application can recommend or dismiss strategic measures on the basis of the KPIs, and different methods within machine learning can be applied. These include logistic regression, k-nearest neighbors, neural networks, or support vector machines. It should be noted here that KPI data, as well as data on strategies taken, must be available for as long as possible at branch level. Ideally, this data should have been compiled correctly and without any gaps, as each model can only be trained as good as the underlying data allows.
Once the model has been trained using the data, the first step is to test a data record and determine the quality of the results. If the model’s performance based on unknown data is good enough, the model has “learned” which strategic measures can be derived from certain combinations of KPIs. In the next step, the model is “fed” current KPI data. This allows calculation of the appropriate strategic measures, which are then submitted to the Management Board to aid decision-making.
The Management Board saves a considerable amount of time, as these measures are presented without a need to interpret data. After each control cycle, the final strategic measures taken are used to further train the model. This means continuous improvement of the algorithm’s performance.
Artificial intelligence offers real potential on the way to Smart Data
In theory, the algorithm could be applied to the entire branch management once accuracy of suggested strategic measures approaches 100 percent. It must be prevented from developing new measures “independently” however, and in new situations the control process will need human assistance again since supervised learning systems can only handle information previously taught. Apart from that, a machine that has undergone sufficient training can actually perform standard control processes autonomously, which promises great potential for companies.
Machine learning is the key technology for intelligent systems and offers high potential for companies. The data already exists, now it is important to use it in the desired correlation. Companies that recognize this opportunity are well on the right path to using their data in a "smart" way.
The article is also published on LinkedIn.