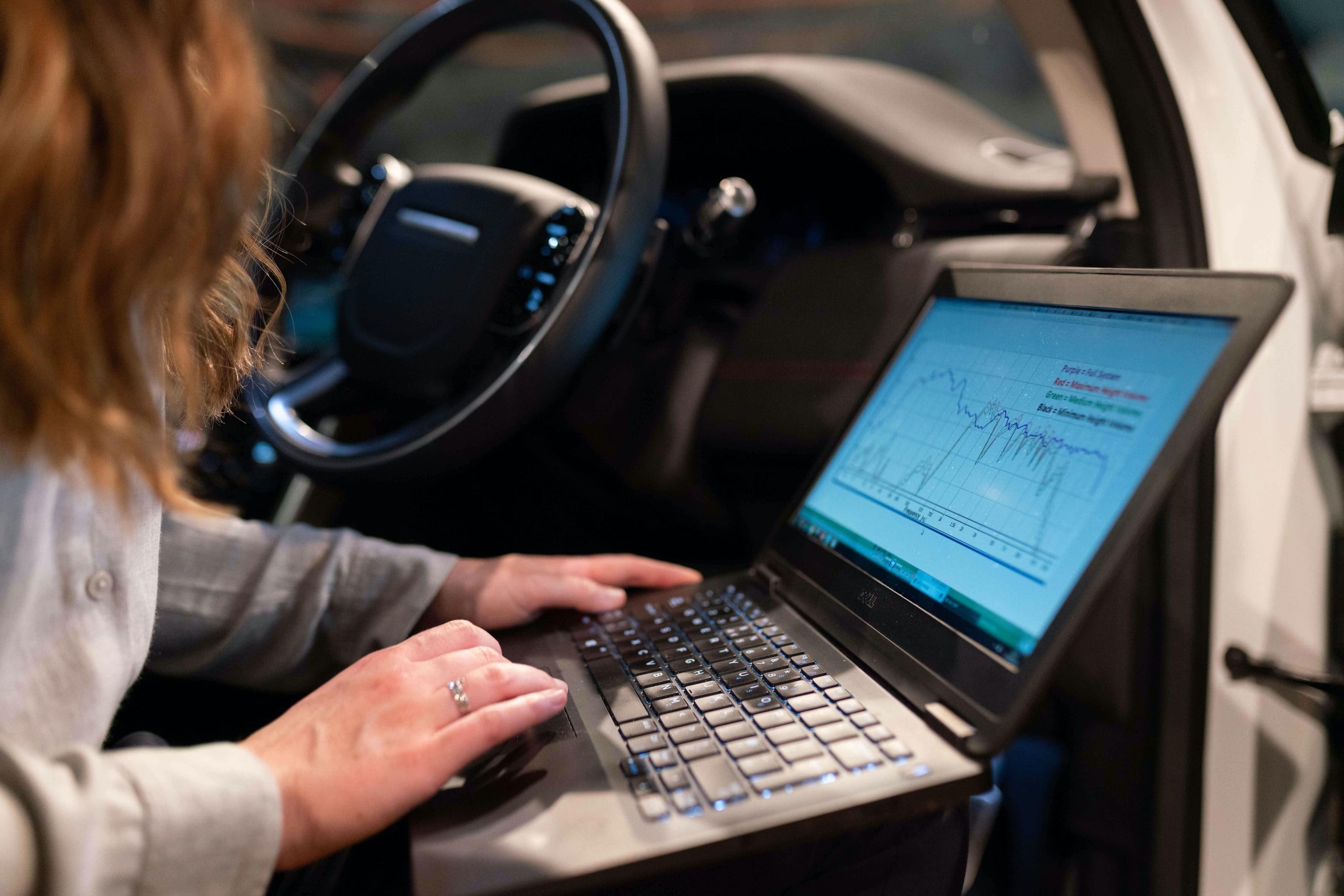
Automotive Aftersales: Top 5 Data Analytics Use Cases
Automotive Aftersales in the Stream of Digitalization and Automation
The advancing digital transformation and in particular AI & automation are changing the future of almost all industries. Automotive aftersales is also continuously facing new challenges such as a decreasing customer loyalty, high competitive pressure, low margins, shortage of skilled labor and the customer expectation of a "seamless online-offline experience".
However, digitization does not only pose risks, but also offers opportunities for lower costs, higher profits and to stand out from the competition - provided that data-driven use cases with a real added value for the workshop and the customer are identified. In the following, we would like to introduce you to 5 such use cases.
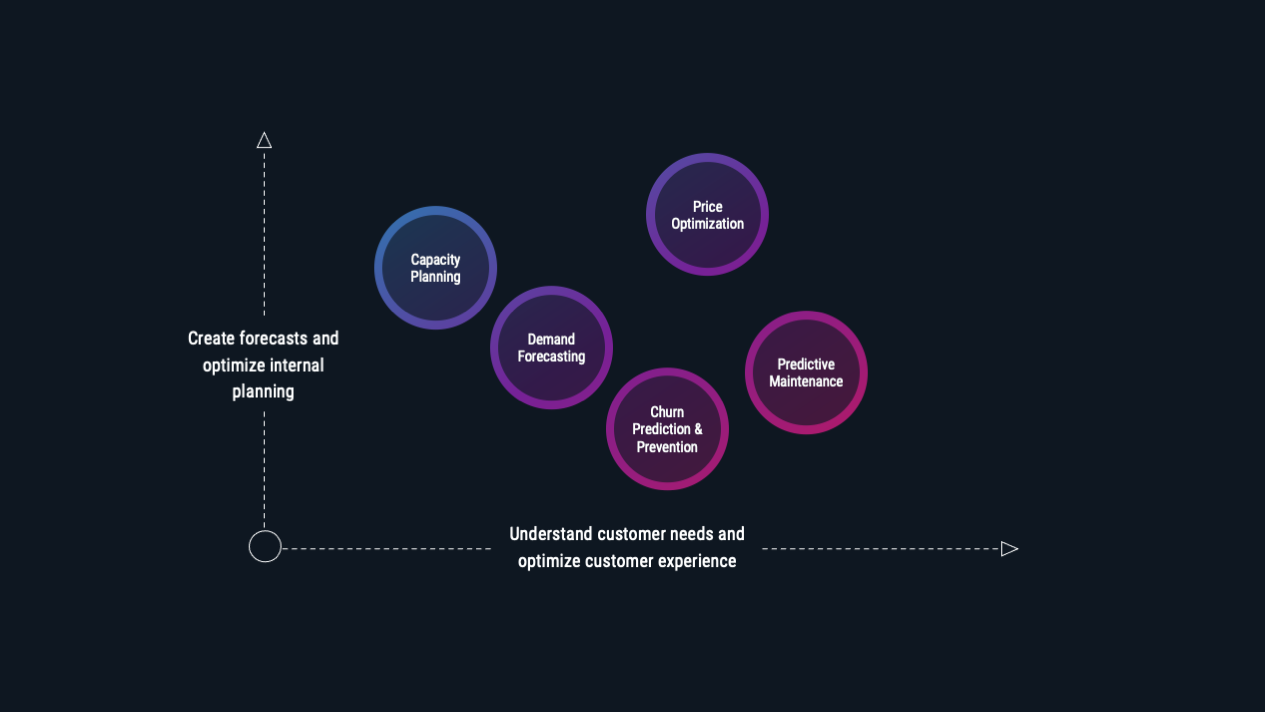
Demand Forecasting
Demand forecasting aims to analyze historical demand over time and, based on this, to make forecasts about future demand. In doing so, the two core questions of which services will be booked when and, which spare parts will be needed when, will be answered.
In practice, the answers to these questions are usually found only in manual planning, controlling reports for the past few months, and estimates of rough market potential. Spare parts are ordered in a similar way every year and, if necessary, stocked.
With a data-driven demand forecast as a basis for decision-making, a workshop can align its services much better with the expected demand - for example, order spare parts on time and in sufficient quantities or keep enough staff on standby for peak times.
Capacity Planning
The aim of capacity planningis to manage the availability of personnel and spare parts in such a way that the long-term demand for services and spare parts can be met as cost-effectively as possible - above all using methods of workforce scheduling and predictive ordering.
The decisive added value of capacity planning lies in the better utilization of existing capacities and in the avoidance of shortages or surpluses of personnel and spare parts. For example, short-term and expensive reordering can be avoided.
Predictive Maintenance
In automotive aftersales, customers usually book a service appointment for the repair or replacement of a vehicle part only when damage occurs. This is often accompanied by the fact that the vehicle is no longer under warranty when the damage occurs. This causes frustration for the customer, which could have been avoided with a timely maintenance.
Predictive maintenance is the practice of proactively approaching customers with maintenance recommendations before a damage occurs. This is accomplished by first calculating failure probabilities for specific vehicle parts using vehicle and vehicle usage data, and then identifying relevant services that dramatically reduce failure occurrences.
By preventing damage in advance, predictive maintenance helps save money over the entire life cycle of a vehicle - as long as damage is covered by the manufacturer's warranty, primarily for the workshops, and subsequently primarily for the customers. Ultimately, this also leads to higher a customer satisfaction and thus loyalty.
Churn Prediction & Prevention
The reasons for churn in automotive aftersales are complex and customer-specific. They can depend, for example, on vehicle type, satisfaction and personal characteristics. Since it is rare for a lost customer to return, it is all the more important to learn from former customers and to prevent the loss of further ones.
Churn prediction & prevention aims to identify customers who are likely to churn at an early stage and to retain them by taking appropriate measures. With booking details and customer master data already available, forecasts can usually be made as to which customers are at risk of churn at what point in time and to what extent. In a second step, measures to prevent churn can be tested based on customer segments or even on a customer-specific basis. In this way, existing customers can be retained instead of having to spend more money on acquiring new customers.
Price Optimization
Demand in the aftersales business is subject to strong fluctuations throughout the year. There are seasonal peaks, for example in the tire business around Easter and October, but before and after that, also periods of no demand are quite common. As digitization progresses, the situation is complicated by the fact that price-sensitive customers are looking for the best deal online.
The aim of price optimization is to influence short- to medium-term demand for services by setting prices for individual customers in such a way that they are distributed evenly over time and the available working time can be used to maximize profits - this procedure is also known as demand smoothing. The first step is to make it possible to offer one and the same core service (e.g., a tire change) at several prices, e.g., through early booking discounts, price surcharges during peak times or discounts during off-peak times. In this way, price optimization can
- win (back) price-sensitive customers by offering attractive prices at off-peak times
- more demand can be met through better utilization of capacities
- different willingness-to-pay can be skimmed off, which means that even higher average prices can be achieved overall.
Our recommendation
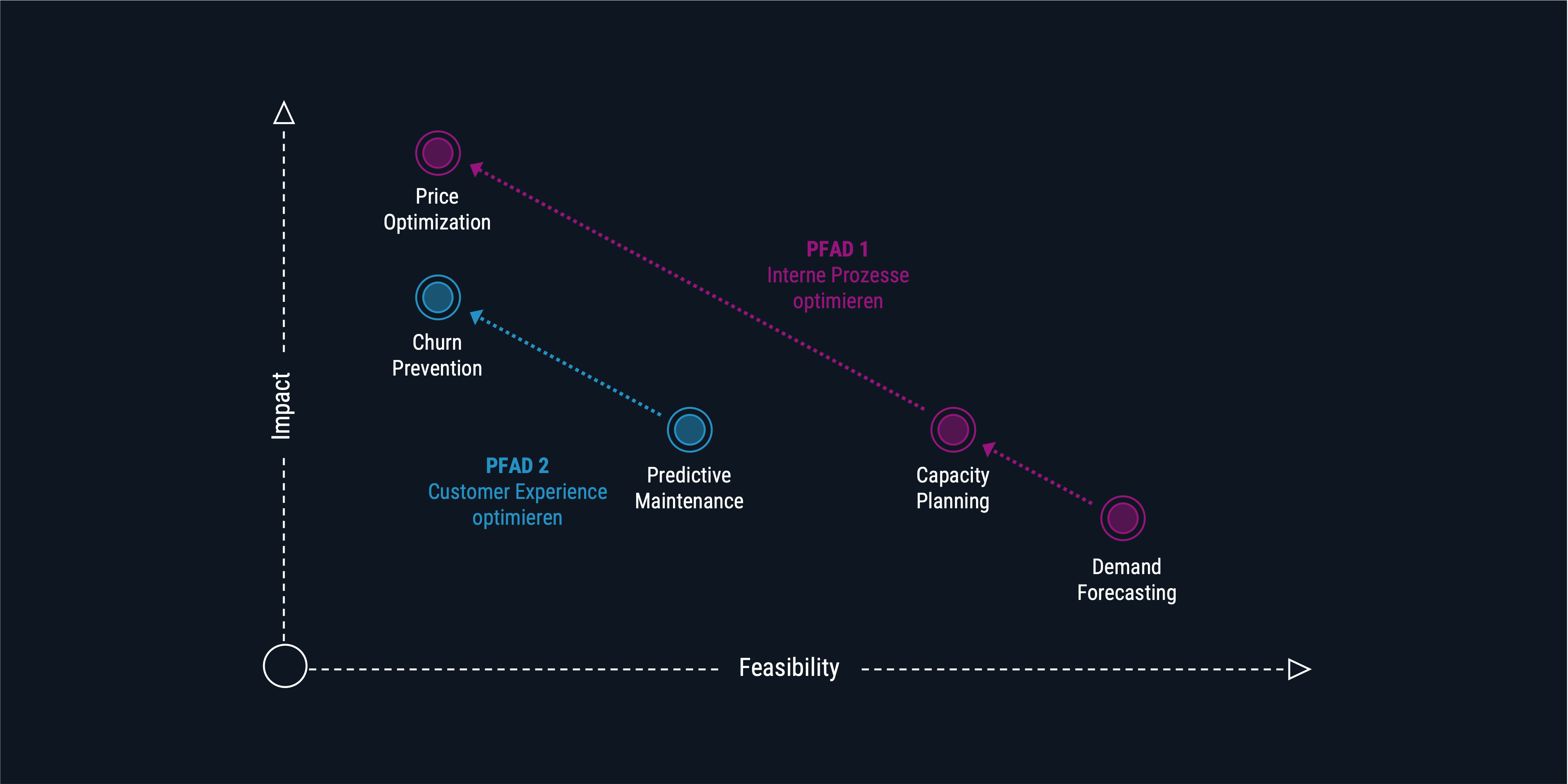
In order to make a recommendation on the order in which the use cases should be considered for implementation, in our experience it is worth taking a look at the feasibility, even if the immediate added value of some other use cases may appear greater at first glance. The following key questions are central:
- For which use cases is data already available?
- Which use cases can be implemented with little effort or are not very complex?
- For which use cases do initial exploratory analyses already provide added value?
- Are there dependencies between the use cases?
Since the collection, integration, and use of customer data are considered sensitive, we recommend starting with the optimization of internal processes. For this purpose, demand forecasting offers the perfect starting point: the necessary data is usually available, explorative analyses are already helpful, and many standard methods are established.
Both capacity planning and price optimization build on successful demand forecasting. Of these two, we recommend continuing with capacity planning, as it mainly only impacts internal stakeholders. Price optimization is much more complex and the necessary data must be generated through extensive trial & error. For example, it is necessary to try out different prices for services randomly in test markets in order to learn the effect of price changes on customer demand.
If the focus is primarily on optimizing the customer experience, we recommend starting with predictive maintenance, as the vehicle and vehicle usage data required for this is already available or easier to obtain. In comparison, the customer-specific data required for churn prediction & prevention, which allows a statement to be made on churn behavior, can be sensitive and is more difficult to obtain.
Have we sparked your interest?
Would you like to discuss the topic with us in more detail?
Get in touch with our rpc contacts!
We are looking forward to speaking with you!