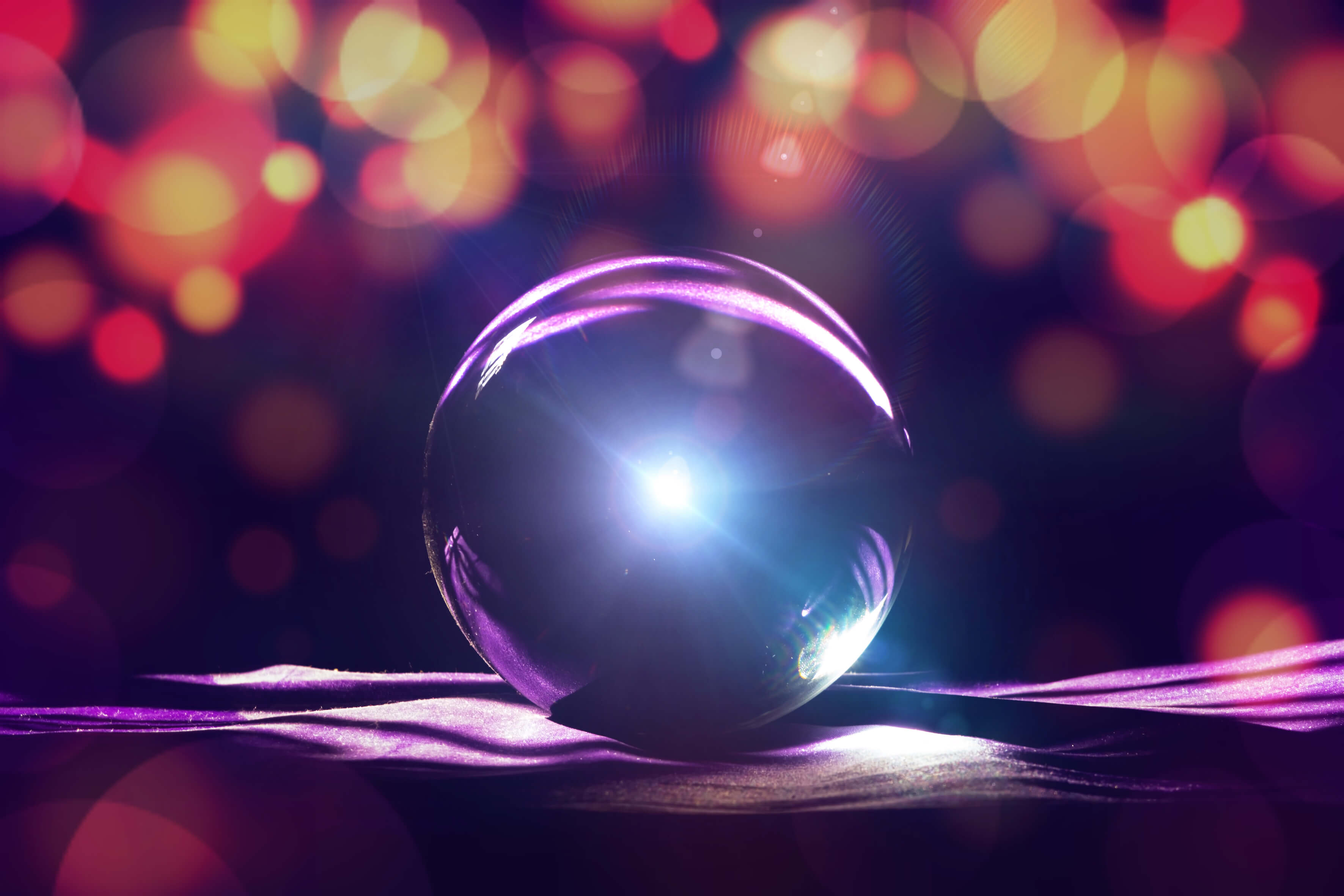
gazing into the future with predictive analytics
by dr. elena michel
The world is becoming more complex than it used to be. Different procedures for statistical data analysis have been around for a long time. The first neuronal learning rule, for example, was written in 1949, and significant developments followed in the mid-1980s. But how can we apply predictive analytics to leverage business performance?
More efficiency with predictive analytics
In recent years, not only have the further development of statistical modeling and computing capacities improved, but also the amount of available data has increased tremendously: Every second, 2,768,150 emails and 8,362 tweets are sent; 77,336 YouTube videos, 1,485 Tumblr posts and 895 Instagram posts are uploaded; 72,275 Google searches are performed; and more than 7 megabytes of data are sent to the cloud by connected cars.
A manual evaluation of these data volumes is very time-consuming and inefficient. With the help of predictive analytics solutions, manual processes can be digitalized and simplified. For example, the prediction of sales figures based on Excel can be replaced by automated predictive analytics solutions with appropriate tools. This use case can provide companies with a great improvement in their contribution margin: They can save up to €70 million per year via automated forecasts.
This is just one example of how predictive analytics enhances the value chain and makes your business more efficient. Across the whole value chain from research & development to supply chain & logistics to marketing & sales as well as within internal domains such as human resources or finance & controlling (see Figure 1), we see many analytical use cases:
Figure 1: Predictive analytics across the value chain
In the following, we have a closer look at four of them: procurement analytics, quality analytics, customer analytics and HR analytics.
Procurement analytics
Procurement managers use predictive analytics to uncover unknown patterns and relationships within complex data sets in order to optimize contract management, supplier evaluation and segmentation or demand forecasting.
Supplier evaluation
The increase in adherence to delivery dates and the ability to deliver is regarded as a great challenge in supplying customers on time. Companies create a 360° view of supplier performance in terms of price, quality and time via intelligent scoring of suppliers based on historical data from internal and external sources. An assessment of corporate social responsibility and the suppliers’ ecological awareness takes place with web scraping, social media analytics and the analyses of external data like environmental certifications or supplier databases. Predictive analytics methods are used to forecast supplier behavior and products or service quality like the risk of underperformance or supply shortage.
Demand forecasting
Companies strive for an optimal balance between the supply of goods at the point of sale and the lowest possible tie-up of capital due to stockpiling. Automotive companies deploy predictive analytics to forecast short-, mid- and long-term demand for models, engines, option take rates or original spare parts per country and also use it for demand planning. External effects and market influences like purchasing behavior in the various regions and the availability and costs of raw materials are considered to optimize production capacities according to the market development. Improved stock keeping generates further savings.
Fraud detection and prevention
Companies identify anomalies in sourcing practice and suppliers’ behavior, applying machine learning as well as process mining to anticipate critical situations at the right time. An early warning system based on web scraping and social listening can be established to better react to external effects such as regulations or macroeconomic developments. The automation of manual processes saves time and money: Text mining allows you to screen contracts or proposals faster and more completely, classification models preselect suppliers, and a data-driven identification of critical products ensures the quality of your own products.
Contract management and negotiation
Spend data get analyzed to create synergies in global procurement processes in terms of bundling in global sourcing that provides economies of scale. The bargaining position can be improved by recalculating supplier prices and using balance data of suppliers and their competitors to better understand margins and negotiation ranges. Companies are able to measure the success of their suppliers’ services in marketing and communications based on attribution models and pay them accordingly.
Quality analytics
Quality management has been a relevant topic in the manufacturing industry for years. This concerns process quality in manufacturing as well as product quality and service quality for product-related and customer services. Companies can detect defects or complaints at an early stage to avoid negative business impacts by deploying predictive analytics.
Field analytics
Within the automotive industry, selective and time-consuming manual analyses are performed by field analysts and the warranty department to find and cluster defects and complaints. The use of predictive analytics methods like classification, clustering, forecasting, alerting or text mining enables the fast and automatic detection of the main faults and complaints as well as the early identification of potentially significant negative effects like warranty costs or reputational damage.
Predictive maintenance
Manufacturing companies have to consider maintenance intervals and thus downtime for their production machines. These systems must be serviced before the quality of the product suffers. By collecting and analyzing machine runtime data, companies are able to predict when maintenance will be due, and thus when downtime will occur, and better include it in production planning. Machine maintenance is therefore carried out according to demand – not too early, but above all not too late.
Customer analytics
Companies are continuously looking for ways to use their sales and marketing activities as efficiently as possible. Instead of sending emails to every customer, they use predictive analytics to segment customers according to target groups more precisely and to address them, taking into account purchase probabilities and personal preferences. With the usage of time series and machine learning algorithms automated, granular and accurate forecasts can be made.
Customer value analysis
Companies apply predictive analytics to find the most promising customers for sales promotions. Instead of relying solely on existing customer data like age and purchase history, advanced analytics methods help to determine which customers are particularly worth the effort because they promise comparatively higher sales rates or are more likely to agree to an offer.
Churn prediction
In addition to winning new customers, maintaining customer relationships is indispensable. This includes preventing possible customers from churning. Companies use predictive analytics to forecast the probability of a contractual relationship being terminated on the basis of customer behavior patterns, combining customer master data, transaction data (e.g. purchasing or contact history, complaints and requests), dealer data like leads or offers, market data or external data such as online and social media. The companies then make targeted offers to customers who are highly likely to churn.
Sales forecasts
Automotive companies use data-driven approaches that enable themselves to deliver automated, granular and accurate forecasts that include scenarios with volatile markets and regulatory environments. Based on time series models like Holt-Winters, ARIMA, ARIMAX or VAR and machine learning algorithms like random forest or regression tree, various data sources can be analyzed and assessed. These data sources can be historical data such as registrations or sales data, launch data or macroeconomic data.
Voice of the customer
The large amount of feedback and comments from customers via online and offline channels makes it difficult to have a clear understanding of customer needs. With the use of natural language processing techniques, like classification, keyword extraction or sentiment analysis, faster and fully automated feedback analysis is possible. Companies receive granular insights into the pain points and root causes of negative customer feedback and are able to react faster.
HR analytics
More and more companies use predictive analytics in the area of human resources. Which absolutely makes sense, as the aim of HR analytics is to better understand the interdependencies within the workforce and to forecast (future) employees’ behavior in terms of employee attraction, employee retention, employee development, the offering and use of training or personnel demand analyses.
Recruiting
Predictive analytics helps companies to identify the channels that promise the best talents. The automated prescreening of résumés via natural language processing and text mining is a useful addition to this process and helps to minimize manual effort.
Labor market analyses
With predictive analytics, HR managers view the supply and demand for specific job groups in the market and obtain benchmarks. This can be invaluable for agile companies that need to quickly qualify in new areas in the event of a shortage of supply. If the supply becomes scarce, they can take creative paths to close the gaps, for example by paying more attention to personality profiles than to professional competence.
Predictive demand analytics
Manpower requirements are specified to determine which and how many vacancies are to be filled with executives in the future. Companies are able to predict time series of planned absent times to identify how many executive positions will be vacant due to retirement, parental leave, etc. to avoid a planning gap if the relevant competencies are not available. Drivers of potential termination can be forecast for early identification of open positions. A specified profile of requirements permits sufficient forward planning for filling vacant positions with appropriate candidates.
Beyond the use cases listed above, there are numerous other areas of application for predictive analytics across the value chain. Besides the use cases, we need to have a look at successfully shaping predictive analytics projects. With the following description, we’d like to give you an initial look at how we guide our customers on their way to a data-driven company.
How to apply predictive analytics projects
1. Concept & data preparation
In the first phase, scoping workshops help to find relevant data sources to specify and clarify customer-centric predictive analytics use cases. To identify these use cases and to assess their value and feasibility, it has proven worthwhile to work with innovative approaches like design thinking. A basic concept defines the analytical purpose in this phase. The next steps include the provision of (test) data, data quality and source checks as well as an initial preparation of the data.
2. Model definition
The second phase comprises the elaboration of a detailed concept for the upcoming analysis and the setup of predictive analytics models. These are based on various state-of-the-art predictive analytics and machine learning methods, such as the following:
- Time series analyses (ARIMA, ARIMAX, VAR, HoltWinters, etc.)
- Regression analyses (logit, probit, etc.)
- Classification methods (decision tree, random forest, etc.)
- Association analyses (shopping basket analysis, etc.)
- Neural networks & deep learning
- Anomaly detection
- Markov chain modeling
- Linear optimization
These methods depend on the specific use case and the aim of the project. We support our customers in the selection of the appropriate analytical tools and offer vendor-independent consulting by using the most suitable data analysis tool – whether open- or closed-source software.
3. Selection of best model
The purpose of the next phase is to select the best model based on the forecast quality, plausibility and validity of the model and to finalize the model. This phase includes an iterative process: The applied models get re-modeled based on extended expert input. To do so, several feedback loops are necessary.
4. Model application & implementation
The fourth phase contains the application of the best predictive analytics models. This means a technical handover to the customer as well as model deployment within the existing IT landscape. With our learning champion approach, we offer our customers accompanying professional data analytics training and coaching for business & IT resources. This guarantees that companies get lasting results and achieve a visible performance boost.
This article is also published on LinkedIn.